An official website of the United States government
Official websites use .gov A .gov website belongs to an official government organization in the United States.
Secure .gov websites use HTTPS A lock ( Lock Locked padlock icon ) or https:// means you've safely connected to the .gov website. Share sensitive information only on official, secure websites.
- Publications
- Account settings
- Advanced Search
- Journal List


Structured Reporting of Lung Cancer Staging: A Consensus Proposal
Vincenza granata, roberto grassi, vittorio miele, anna rita larici, nicola sverzellati, salvatore cappabianca, luca brunese, nicola maggialetti, andrea borghesi, roberta fusco, maurizio balbi, fabrizio urraro, duccio buccicardi, chandra bortolotto, roberto prost, marco rengo, elisa baratella, massimo de filippo, carmelo barresi, stefano palmucci, marco busso, lucio calandriello, mario sansone, emanuele neri, francesca coppola, lorenzo faggioni.
- Author information
- Article notes
- Copyright and License information
Correspondence: [email protected]
Received 2021 Jul 27; Accepted 2021 Aug 27; Collection date 2021 Sep.
Licensee MDPI, Basel, Switzerland. This article is an open access article distributed under the terms and conditions of the Creative Commons Attribution (CC BY) license ( https://creativecommons.org/licenses/by/4.0/ ).
Background: Structured reporting (SR) in radiology is becoming necessary and has recently been recognized by major scientific societies. This study aimed to build CT-based structured reports for lung cancer during the staging phase, in order to improve communication between radiologists, members of the multidisciplinary team and patients. Materials and Methods: A panel of expert radiologists, members of the Italian Society of Medical and Interventional Radiology, was established. A modified Delphi exercise was used to build the structural report and to assess the level of agreement for all the report sections. The Cronbach’s alpha (Cα) correlation coefficient was used to assess internal consistency for each section and to perform a quality analysis according to the average inter-item correlation. Results: The final SR version was built by including 16 items in the “Patient Clinical Data” section, 4 items in the “Clinical Evaluation” section, 8 items in the “Exam Technique” section, 22 items in the “Report” section, and 5 items in the “Conclusion” section. Overall, 55 items were included in the final version of the SR. The overall mean of the scores of the experts and the sum of scores for the structured report were 4.5 (range 1–5) and 631 (mean value 67.54, STD 7.53), respectively, in the first round. The items of the structured report with higher accordance in the first round were primary lesion features, lymph nodes, metastasis and conclusions. The overall mean of the scores of the experts and the sum of scores for staging in the structured report were 4.7 (range 4–5) and 807 (mean value 70.11, STD 4.81), respectively, in the second round. The Cronbach’s alpha (Cα) correlation coefficient was 0.89 in the first round and 0.92 in the second round for staging in the structured report. Conclusions: The wide implementation of SR is critical for providing referring physicians and patients with the best quality of service, and for providing researchers with the best quality of data in the context of the big data exploitation of the available clinical data. Implementation is complex, requiring mature technology to successfully address pending user-friendliness, organizational and interoperability challenges.
Keywords: radiology report, free text report, structured report, lung cancer, computed tomography
1. Introduction
The American Recovery and Reinvestment Act and the Health Information Technology for Economic and Clinical Health Act have indicated that structuring data in health records will lead to an important improvement in patient outcomes [ 1 , 2 ]. Since the radiology report is part of the health record, the current format of free-text reporting (FTR) should be organized and shifted toward structured reporting (SR). The issue of whether all radiological examinations should contain a structured report, and if so, what the actual report structure should be, remains open [ 1 , 2 , 3 ]. According to the European Society of Radiology’s (ESR) paper on SR in radiology [ 1 ], the three main reasons for moving from FTR to SR are quality, data quantification and accessibility. A critical quality improvement dimension resulting from the use of SR is standardization. The use of templates in SR provides a checklist as to whether all relevant items for a particular examination have been addressed. Thanks to this “structure”, the radiology report will also allow the association of radiological data and other key clinical features, leading to a precise diagnosis and personalized medicine. With regards to accessibility, it is known that radiology reports are a rich source of data for research. This allows automated data mining, which may help to validate the relevance of imaging biomarkers by highlighting the clinical contexts in which they are most appropriate, and to devise potential new application domains. For this reason, radiology reports should be structured via their content, based on standard terminology, and should be accessible via standard access mechanisms and protocols.
Weiss et al. have described three levels of SR [ 4 ]:
The first level is a structured format with paragraphs and subheadings. Currently, almost all radiology reports display this structure, with sections for clinical information, examination protocol and radiological findings, and a conclusion to highlight the most important findings.
The second level refers to consistent organization. For example, rectal cancer magnetic resonance imaging (MRI) describes all relevant features, such as tumor (T) stage, node (N) stage, anal sphincter complex involvement, tumor deposits in the mesorectal space, extramural vascular invasion, etc.
The third level directly addresses the consistent use of dedicated terminology, namely, standard language.
Several proposals have been made by major International Societies of Radiology to support the use of SR [ 5 , 6 , 7 , 8 , 9 , 10 ]. The Italian Society of Medical and Interventional Radiology (SIRM) has created an Italian warehouse of SR templates, which can be freely accessed by all SIRM members, for the purpose of being routinely used in a clinical setting [ 11 ].
Despite these promising developments, SR has not yet been established in clinical routine. A survey of Italian radiologists found that the majority of those surveyed had heard of SR, but only a minority of them regularly used it in their clinical work [ 10 ]. Reasons for this include the current lack of usable templates and the minimal availability of software solutions for SR [ 10 ].
Lung cancer is the leading cause of cancer morbidity and mortality in men, whereas in women, it ranks third for incidence after breast and colorectal cancer, and second for mortality after breast cancer [ 12 ]. The incidence and mortality rates are roughly twice as high in men than in women, although the male-to-female ratio varies widely across regions. Lung cancer incidence and mortality rates are 3 to 4 times higher in transitioned countries than in transitioning countries; this pattern may well change as the tobacco epidemic evolves, given that 80% of smokers aged 15 years or older resided in low-income and middle-income countries in 2016 [ 12 , 13 ]. In the absence of symptoms to identify early lung cancer, screening high-risk individuals has the potential of shifting the diagnosis to earlier stages [ 14 , 15 , 16 , 17 , 18 , 19 , 20 ]. After more than 30 years of research, a large randomized controlled trial established that low-dose computed tomography (CT) improved mortality in patients at high risk for lung cancer. Subsequently, the majority of professional societies emphasize the importance of lung cancer screening. Although lung cancer screening is not unanimously recommended, the value of identifying early-stage lung cancer cannot be overstated. The majority of new cases of lung cancer present in advanced stages (III–IV), when a cure is unlikely or unattainable [ 21 ].
In this context, a disease-specific SR could be an effective tool for conveying all diagnostic imaging information needed for a correct lung cancer diagnosis and staging, while including clinical information required for personalized patient management.
The aim of the present study was to propose an SR template that can guide radiologists in the systematic reporting of CT examinations for lung cancer staging, in order to improve communication between radiologists and clinicians, particularly in non-referral centers.
2. Materials and Methods
2.1. panel expert.
As a result of critical discussion between expert radiologists, a multi-round consensus-building Delphi exercise was carried out to develop a comprehensive and focused SR template for CT staging of patients with lung cancer.
A SIRM radiologist expert in thoracic imaging created the first draft of the SR template for lung cancer staging CT examinations.
A working team of 13 experts from the Italian College of Thoracic Radiologists and of Diagnostic Imaging in Oncology Radiologists from SIRM was established to iteratively revise the initial draft, with the aim of reaching a final consensus on SR.
2.2. Selection of the Delphi Domains and Items
All the experts reviewed the literature data on the main scientific databases (including Pubmed, Scopus and Google Scholar) from December 2000 to December 2020, in order to assess papers on lung cancer CT and radiological SR. The full text of the studies selected was reviewed by all members of the expert panel, and each of them developed and shared the list of Delphi items via email and/or teleconference.
The SR was divided into five sections: (1) Patient Clinical Data, (2) Clinical Evaluation, (3) Exam Technique, (4) Report and (5) Conclusion. A dedicated section for key images was added as part of the report.
The “Patient Clinical Data” section included patient clinical data and previous or family history of malignancies, including previous lung cancer, risk factors or predisposing pathologies. In this section, the item of “Allergies” to drugs and contrast medium was included.
The “Clinical Evaluation” section included previous examination results, a genetic panel and clinical symptoms.
The “Exam Technique” section included data regarding the CT equipment used (including the number of detector rows and whether single or dual energy scans were performed) and information concerning reconstruction algorithm(s) and slice thickness. Data on the contrast protocol were also collected (including information regarding post-contrast acquisitions), as well as data concerning the contrast medium (such as contrast active principle, commercial name, volume, flow rate, iodine concentration, and ongoing adverse events).
The “Report” section included data regarding lung cancer location, morphology, margin sharpness, texture (e.g., solid, ground glass), contrast enhancement pattern, size, local invasion, tumor stage, node stage and metastatic stage, according to the Italian Association of Medical Oncology (AIOM) guidelines [ 22 ]. In this section, a dedicated subsection for other types of primary lung cancers was included.
The “Conclusion” section included diagnosis, TNM stage according to the 8th Edition of AJCC-UICC 2017 [ 23 ], annotations and comments.
Two Delphi rounds were carried out [ 24 ]. During the first round, each panelist independently contributed to refining the SR draft by means of online meetings or email exchanges. The level of panelist agreement for each SR model was tested in the second Delphi round, using a Google Form questionnaire shared by email. Each expert made individual comments for each specific template part (i.e., patient clinical data, clinical evaluation, exam technique, report and conclusion, images) using a five-point Likert scale (1 = strongly disagree, 2 = slightly disagree, 3 = slightly agree; 4 = modestly agree, 5 = strongly agree).
After the second Delphi round, the final version of the SR was generated on the dedicated Radiological Society of North America (RSNA) website (radreport.org), using a T-Rex template format in line with the IHE (Integrating the Healthcare Enterprise) and MRRT (Management of Radiology Report Templates) profiles, accessible as open-source software, with the technical support of Exprivia™. These determined both the format of the radiology report templates (using version 5 of the Hypertext Markup Language (HTML5)) and the transporting mechanism used to request, get back and stock these schedules [ 25 ]. The radiology report was structured using a series of “codified queries” integrated into the T-Rex editor’s preselected sections [ 25 ].
2.3. Statistical Analysis
All ratings of the panelists for each section were analyzed using descriptive statistics measuring the mean score, the standard deviation value (STD) and the sum of scores. A mean score of 3 was considered good and a score ≥ 4 excellent.
To measure the internal consistency of the panelist ratings for each section of the report, a quality analysis based on the average inter-item correlation was carried out using the Cronbach’s alpha (Cα) correlation coefficient [ 26 , 27 ]. The Cα test provides a measure of the internal consistency of a test or scale; it is expressed as a number between 0 and 1. Internal consistency describes the extent to which all the items in a test measure the same concept. The Cα correlation coefficient was determined after each round.
The closer to 1.0 the Cα coefficient, the greater the internal consistency of the items in the scale. An alpha coefficient (α) > 0.9 was considered excellent, α > 0.8 good, α > 0.7 acceptable, α > 0.6 questionable, α > 0.5 poor, and α < 0.5 unacceptable. However, in the iterations, an α of 0.8 was considered to be a reasonable goal for internal reliability.
The data analysis was carried out using Statistic Toolbox of Matlab (The MathWorks, Inc., Natick, MA, USA).
3.1. Structured Report
The final SR ( Appendix A ) version was built by including 16 items in the “Patient Clinical Data” section, 4 items in the “Clinical Evaluation” section, 8 items in the “Exam Technique” section, 22 items in the “Report” section, and 5 items in the “Conclusion” section. Overall, 55 items were included in the final version of the SR. In Appendix B , the first draft of the SR is illustrated.
The results obtained during the first Delphi round are reported in Table 1 , and those obtained after the second Delphi round in Table 2 .
Single scores and the sums of scores of panelists for staging in the SR (round I).
Note STD: standard deviation value; CM: contrast medium.
Single scores and sums of scores of panelists for staging in the SR (round II).
In the final version of the SR, the following parameters were included:
In the “Exam technique” section, the equipment used, the number of detector rows and CT modality (i.e., single or dual energy), the reconstruction algorithm(s) used and contrast protocol;
In the “Report” section, the sites and the features of extrathoracic metastases were defined, identifying the target lesions in accordance with the Response Evaluation Criteria in Solid tumors (RECIST) 1.1 [ 28 ].
3.2. Consensus Agreement
Table 1 and Table 2 show the single scores and the sums of scores of the panelists for staging with the SR in the first and second rounds, respectively.
In both the first and the second rounds, as reported in Table 1 and Table 2 , all sections received more than a good rating.
The overall mean score of the experts (13 experts) and the sum of scores for staging with the SR were 4.5 (range 1–5) and 631 (mean value 67.54, STD 7.53) ( Table 1 ), respectively, in the first round. The items of the SR with higher accordance in the first round were primary lesion features, lymph nodes, metastases and conclusions ( Table 1 ).
The overall mean score of the experts (nine experts) and the sum of scores for staging with the SR were 4.7 (range 4–5) and 807 (mean value 70.11, STD 4.81) ( Table 2 ), respectively, in the second round.
The overall mean score of the experts in the second round was higher than the overall mean score of the experts in the first round, with a lower standard deviation value demonstrating the higher agreement reached among the experts in the SR in this round. The items of the SR in the second round that had higher “each reader” accordance were exam data and pulmonary involvement in multiple sites ( Table 2 ).
The Cronbach’s alpha (Cα) correlation coefficient was 0.89 in the first round and 0.92 in the second round for staging with the SR.
4. Discussion
In the present study, the panel of experts demonstrated a high degree of agreement in defining the different items of the SR. After the second Delphi round, the panelists’ mean score and the sum of scores related to the SR models were 4.7 (range 4–5) and 807 (mean value 70.11, STD 4.81), respectively. All sections received more than a good rating in the second Delphi round; however, the weakest sections were “Patient Clinical Data” and “Clinical Evaluation”. Moreover, the Cα correlation coefficient reached 0.92 in the second round.
The present SR is based on a multi-round consensus-building Delphi exercise performed to develop a comprehensive focus on the SR template for CT-based lung cancer staging, as a result of critical discussion between expert radiologists in thoracic and oncological imaging. This SR was based on a standardized terminology and structure, which are aspects required for adherence to diagnostic-therapeutic recommendations and for enrolment in clinical trials, thus reducing the ambiguity that may arise from non-conventional language, and enabling better communication between radiologists and clinicians [ 29 , 30 , 31 , 32 , 33 ]. Therefore, according to Weiss et al. [ 4 ], the present report is a third-level SR.
Several sections are included in the present template: “Patient Clinical Data”, “Clinical Evaluation”, “Exam Technique”, “Report” and “Conclusion”. Some points should be evaluated for each of these sections.
Regarding “Patient Clinical Data”, this section included data regarding personal or family history of cancer, and exposure to different risk factors and any genetic mutations. Regarding predisposing diseases, the possibility of collecting data on Chronic Obstructive Pulmonary Disease (COPD) allows one to plan treatment tactics. COPD is generally defined as a chronic minimally reversible airflow obstruction based on spirometry (post-bronchodilator forced expiratory volume in 1 second (FEV1)/forced vital capacity (FVC) less than 70%).
COPD and lung cancer share common features, including their high mortality and common risk factors (such as smoking), some genetic background, environmental exposures, and underlying common inflammatory processes. A ratio of FEV1 to FVC less than 0.7 is generally used to define airflow obstruction; however, other indices (such as FEV1/FVC under the lower limit of normal criteria, and a predicted reduction of FEV1%) have also been considered indicative of airway obstruction. In addition to these three main factors, the timing of COPD diagnosis, the degree of airflow obstruction, and the severity of emphysema have also been reported to exert a remarkable effect on the significance of the impact of COPD and/or emphysema on lung cancer risk. Although, at present, no solid evidence is available to clearly distinguish the roles of airflow obstruction and emphysema in lung cancer development, it is certain that the highest lung cancer risk occurs when airflow obstruction and emphysema coexist [ 12 , 13 , 14 ].
Such a painstaking process of data collection was subject to some disagreement among the panelists due to the opinion that this process could slow down the normal workflow and was not considered to be easy to use. However, it is necessary to point out that all SR sections are independent from each other, so that the Patient Clinical Data and Clinical Evaluation sections are optional and may be filled in or not upon user choice, although they were conceived with the aim of creating databases. In fact, the possibility of collecting all these data could allow the creation of a large database, not only for epidemiological studies, but also in the highest conception of radiology, to lay the foundations for radiomics studies [ 34 , 35 , 36 , 37 ]. Radiology reports should be rich in data that could potentially be pooled, analyzed and correlated with patient outcomes, thereby assisting future clinical and imaging guidelines. However, the use of non-standardized terminology limits the capacity for data collection across multiple institutions. In addition, the lack of consistent data extractable from SR could hinder the development of computerized applications to assist in reporting. Natural language processing applications can help extract the data from the reports with variable terminology, allowing the compilation of standardized data, which could then be used to develop multi-institutional data registries, as well as in clinical and research analyses. Moreover, the possibility of combining genomic data and radiological features allows for developing models of radiogenomics—models that today represent the highest level of advanced-precision medicine processes [ 38 , 39 , 40 , 41 ]. The fact that the present SR can be included in the picture archiving and communication system (PACS) is an added value; therefore, it is only necessary to enter these data once upon first entry into the radiology department.
With regard to the “Exam Technique” section, sharing the examination technique not only within one’s own department, but also with the radiology departments of other centers, fulfills a dual purpose. On the one hand, it enables the standardization of CT protocols; on the other hand, it allows carrying out diagnostic accuracy studies among different centers in order to optimize CT protocols. For example, during follow-up, differences in CT acquisition parameters and segmentation algorithms are important factors that can lead to variability in volumetric measurements. Therefore, slice thickness and other protocol-related factors (such as the reconstruction kernel and field of view) should be kept constant for reliable measurements to be carried out. Although some software packages allow the customization of options (which changes density thresholds for segmentation), standardized parameters should exist between practices in order to keep these parameters homogenous and comparable. In the CT protocol optimization step, enhanced communication among different centers could theoretically lead to quality improvement by means of enhanced patient safety (e.g., by radiation dose reduction), contrast optimization, and image quality. With improved communication comes the sharing of knowledge and experience, along with the potential of reducing medical errors and improving clinical outcomes [ 42 ].
Some authors have reported that the use of a checklist could improve diagnostic accuracy [ 43 , 44 , 45 ]. In 2014, based on the results of several screening trials, the American College of Radiology (ACR) released version 1.0 of the Lung CT Screening Reporting and Data System (Lung-RADS) [ 46 ]. This is a standardized method of reporting with recommendations for the management of pulmonary nodules detected on CT for lung cancer screening. When utilized, it can reduce the false positive rate in lung cancer screening, without increasing the rates of false negatives [ 47 , 48 ]. Lung-RADS is now deeply embedded as a quality metric on which regulation and reimbursement is determined by the Centers for Medicare and Medicaid [ 49 , 50 ]. During the first 5 years of nationwide lung cancer screening, there was a significant accumulation of data and experience, with many opportunities for continued learning [ 49 , 50 ].
The present “Report” section was designed to report all the structural characteristics of the lesions, such as margins and density, as well as relationships with locoregional structures (e.g., the pleura), which allow correct staging, but could also impact the choice of a more suitable therapeutic treatment based on the individual patient. The advantages of SR over FTR include its standardized terminology and structure, aspects required for adherence to diagnostic-therapeutic recommendations and for enrolment in clinical trials. SR reduces the ambiguity that may arise from non-conventional language. However, it should be noted that SR templates usually include a free text box for reporting any additional data that cannot be embedded in the default template fields.
The wide implementation of SR is critical for providing referring physicians and patients with the best quality of service, and for providing researchers with best quality data in the context of the big data exploitation of available clinical information [ 51 , 52 , 53 , 54 ]. Implementation is complex, requiring mature technology to successfully address pending user-friendliness, organizational and interoperability challenge (with particular regard to the adequate storage of data, and easy and adequate connections with PACS and post-processing software). Consequently, the introduction of SR should be seen as a comprehensive effort, affecting all domains of radiology [ 55 , 56 , 57 , 58 ].
Despite the promising results obtained, this study has some limitations. First, the panelists were all radiologists; therefore, a multidisciplinary approach is lacking. A multidisciplinary validation of SR would have been more appropriate. Second, the panelists were of the same nationality; contributions from experts from multiple countries would allow for broader sharing, and would increase the consistency of the SR. Finally, this study was not aimed at assessing the impact of SR on the management of patients with lung cancer. This issue will be discussed in forthcoming studies.
5. Conclusions
The wide implementation of SR is a critical point for providing referring physicians and patients with the best quality of service, and for providing researchers with the best quality of data in the context of the big data exploitation of the available clinical information. Implementation is complex, requiring mature technology to successfully address pending user-friendliness, organizational and interoperability challenges (specifically, the adequate storage of data, and the easy and adequate connection with PACS and post-processing software). Consequently, the introduction of SR should be seen as a comprehensive effort, affecting all domains of radiology.
The authors have no conflict of interest to disclose. The authors confirm that the article is not under consideration for publication elsewhere. Each author participated sufficiently to take public responsibility for the content of the manuscript.
Patient’s clinical data (imported from RIS).
Patient’s history.
Clinical evaluation.
Imaging protocol.
ADVERSE EVENTS.
PATIENT’S CLINICAL DATA (imported from RIS).
CLINICAL EVALUATION.
IMAGING PROTOCOL.
Author Contributions
Conceptualization, R.G.; Data curation, V.G. and V.M.; Formal analysis, V.G. and M.S.; Investigation, V.G., R.G., V.M., A.R.L., N.S., S.C., L.B., N.M., A.B., R.F., M.B. (Maurizio Balbi), F.U., D.B., C.B. (Chandra Bortolotto), R.P., M.R., E.B., M.D.F., C.B. (Carmelo Barresi), S.P., M.B. (Marco Busso), E.N., F.C. and L.F.; Methodology, V.G., R.G., V.M., A.R.L., N.S., S.C., M.B. (Maurizio Balbi), F.U., D.B., C.B. (Chandra Bortolotto), M.R., E.B., M.D.F., C.B. (Carmelo Barresi), S.P., M.B. (Marco Busso), L.C., E.N., F.C. and L.F.; Project administration, R.P. All authors have read and agreed to the published version of the manuscript.
This research received no external funding.
Institutional Review Board Statement
Not applicable.
Informed Consent Statement
Data availability statement.
All data are reported in this manuscript.
Conflicts of Interest
The authors declare no conflict of interest.
Publisher’s Note: MDPI stays neutral with regard to jurisdictional claims in published maps and institutional affiliations.
- 1. European Society of Radiology (ESR) ESR paper on structured reporting in radiology. Insights Imaging. 2018;9:1–7. doi: 10.1007/s13244-017-0588-8. [ DOI ] [ PMC free article ] [ PubMed ] [ Google Scholar ]
- 2. American Recovery and Reinvestment Act of 2009–Title XIII: Health Information Technology: Health Information Technology for Economic and Clinical Health Act (HITECH Act) US Government. [(accessed on 20 April 2021)];:112–164. Available online: https://www.healthit.gov/sites/default/files/hitech_act_excerpt_from_arra_with_index.pdf .
- 3. Granata V., Caruso D., Grassi R., Cappabianca S., Reginelli A., Rizzati R., Masselli G., Golfieri R., Rengo M., Regge D., et al. Structured Reporting of Rectal Cancer Staging and Restaging: A Consensus Proposal. Cancers. 2021;13:2135. doi: 10.3390/cancers13092135. [ DOI ] [ PMC free article ] [ PubMed ] [ Google Scholar ]
- 4. Weiss D.L., Bolos P.R. Branstetter IV BF: Practical Imaging Informatics: Foundations and Applications for PACS Professionals. Springer; Heidelberg, Germany: 2019. Reporting and dictation. [ Google Scholar ]
- 5. Persigehl T., Baumhauer M., Baeßler B., Beyer L.P., Bludau M., Bruns C., Bunck A.C., Germer C.-T., Grenacher L., Hackländer T., et al. Structured Reporting of Solid and Cystic Pancreatic Lesions in CT and MRI: Consensus-Based Structured Report Templates of the German Society of Radiology (DRG) RöFo-Fortschr. Auf Dem Geb. Der Röntgenstrahlen Und Der Bildgeb. Verfahr. 2020;192:641–656. doi: 10.1055/a-1150-8217. [ DOI ] [ PubMed ] [ Google Scholar ]
- 6. Beets-Tan R.G.H., Lambregts D.M.J., Maas M., Bipat S., Barbaro B., Curvo-Semedo L., Fenlon H.M., Gollub M.J., Gourtsoyianni S., Halligan S., et al. Magnetic resonance imaging for clinical management of rectal cancer: Updated recommendations from the 2016 European Society of Gastrointestinal and Abdominal Radiology (ESGAR) consensus meeting. Eur. Radiol. 2018;28:1465–1475. doi: 10.1007/s00330-017-5026-2. [ DOI ] [ PMC free article ] [ PubMed ] [ Google Scholar ]
- 7. Neri E., Coppola F., Larici A.R., Sverzellati N., Mazzei M.A., Sacco P., Dalpiaz G., Feragalli B., Miele V., Grassi R. Structured reporting of chest CT in COVID-19 pneumonia: A consensus proposal. Insights Imaging. 2020;11:1–9. doi: 10.1186/s13244-020-00901-7. [ DOI ] [ PMC free article ] [ PubMed ] [ Google Scholar ]
- 8. Simpson S., Kay F.U., Abbara S., Bhalla S., Chung J.H., Chung M., Henry T.S., Kanne J.P., Kligerman S., Ko J.P., et al. Radiological Society of North America Expert Consensus Document on Reporting Chest CT Findings Related to COVID-19: Endorsed by the Society of Thoracic Radiology, the American College of Radiology, and RSNA. Radiol. Cardiothorac. Imaging. 2020;2:e200152. doi: 10.1148/ryct.2020200152. [ DOI ] [ PMC free article ] [ PubMed ] [ Google Scholar ]
- 9. Sverzellati N., Consort T.I.S.R.O.F.L.D., Odone A., Silva M., Polverosi R., Florio C., Cardinale L., Cortese G., Addonisio G., Zompatori M., et al. Structured reporting for fibrosing lung disease: A model shared by radiologist and pulmonologist. La Radiol. Med. 2018;123:245–253. doi: 10.1007/s11547-017-0835-6. [ DOI ] [ PMC free article ] [ PubMed ] [ Google Scholar ]
- 10. Faggioni L., Coppola F., Ferrari R., Neri E., Regge D. Usage of structured reporting in radiological practice: Results from an Italian online survey. Eur. Radiol. 2016;27:1934–1943. doi: 10.1007/s00330-016-4553-6. [ DOI ] [ PubMed ] [ Google Scholar ]
- 11. [(accessed on 20 April 2021)]. Available online: http://www.sirm.org .
- 12. Sung H., Ferlay J., Siegel R.L., Laversanne M., Soerjomataram I., Jemal A., Bray F. Global Cancer Statistics 2020: GLOBOCAN Estimates of Incidence and Mortality Worldwide for 36 Cancers in 185 Countries. CA Cancer J. Clin. 2021;71:209–249. doi: 10.3322/caac.21660. [ DOI ] [ PubMed ] [ Google Scholar ]
- 13. World Health Organization (WHO) Global Health Estimates 2020: Deaths by Cause, Age, Sex, by Country and by Region, 2000–2019. WHO; Geneva, Switzerland: 2020. [ Google Scholar ]
- 14. Fusco R., Granata V., Mazzei M.A., Di Meglio N., Del Roscio D., Moroni C., Monti R., Cappabianca C., Picone C., Neri E., et al. Quantitative imaging decision support (QIDSTM) tool consistency evaluation and radiomic analysis by means of 594 metrics in lung carcinoma on chest CT scan. Cancer Control. 2021;28 doi: 10.1177/1073274820985786. [ DOI ] [ PMC free article ] [ PubMed ] [ Google Scholar ]
- 15. Agazzi G.M., Ravanelli M., Roca E., Medicina D., Balzarini P., Pessina C., Vermi W., Berruti A., Maroldi R., Farina D. CT texture analysis for prediction of EGFR mutational status and ALK rearrangement in patients with non-small cell lung cancer. La Radiol. Med. 2021;126:786–794. doi: 10.1007/s11547-020-01323-7. [ DOI ] [ PubMed ] [ Google Scholar ]
- 16. Kim B.H., Kim J.-S., Kim K.H., Moon H.J., Kim S. Clinical significance of radiation dose–volume parameters and functional status on the patient-reported quality of life changes after thoracic radiotherapy for lung cancer: A prospective study. La Radiol. Med. 2021;126:466–473. doi: 10.1007/s11547-020-01273-0. [ DOI ] [ PubMed ] [ Google Scholar ]
- 17. Danti G., Berti V., Abenavoli E., Briganti V., Linguanti F., Mungai F., Pradella S., Miele V. Diagnostic imaging of typical lung carcinoids: Relationship between MDCT, 111In-Octreoscan and 18F-FDG-PET imaging features with Ki-67 index. La Radiol. Med. 2020;125:715–729. doi: 10.1007/s11547-020-01172-4. [ DOI ] [ PubMed ] [ Google Scholar ]
- 18. Farchione A., Larici A.R., Masciocchi C., Cicchetti G., Congedo M.T., Franchi P., Gatta R., Cicero S.L., Valentini V., Bonomo L., et al. Exploring technical issues in personalized medicine: NSCLC survival prediction by quantitative image analysis—usefulness of density correction of volumetric CT data. La Radiol. Med. 2020;125:625–635. doi: 10.1007/s11547-020-01157-3. [ DOI ] [ PubMed ] [ Google Scholar ]
- 19. Zhang G., Yang Z., Gong L., Jiang S., Wang L., Zhang H. Classification of lung nodules based on CT images using squeeze-and-excitation network and aggregated residual transformations. La Radiol. Med. 2020;125:374–383. doi: 10.1007/s11547-019-01130-9. [ DOI ] [ PubMed ] [ Google Scholar ]
- 20. Franceschini D., Bruni A., Borghetti P., Giaj-Levra N., Ramella S., Buffoni L., Badellino S., Andolina M., Comin C., Vattemi E., et al. Is multidisciplinary management possible in the treatment of lung cancer? A report from three Italian meetings. La Radiol. Med. 2019;125:214–219. doi: 10.1007/s11547-019-01094-w. [ DOI ] [ PubMed ] [ Google Scholar ]
- 21. Bade B.C., Brasher P.B., Luna B.W., Silvestri G.A., Tanner N.T. Reviewing Lung Cancer Screening. Clin. Chest Med. 2018;39:31–43. doi: 10.1016/j.ccm.2017.09.003. [ DOI ] [ PubMed ] [ Google Scholar ]
- 22. [(accessed on 20 April 2021)]. Available online: https://www.aiom.it/linee-guida-aiom-2020-neoplasie-del-polmone/
- 23. [(accessed on 20 April 2021)]. Available online: https://www.uicc.org/news/8th-edition-uicc-tnm-classification-malignant-tumors-published .
- 24. Dalkey N., Helmer O. An Experimental Application of the DELPHI Method to the Use of Experts. 1963. [(accessed on 21 January 2021)]. Available online: https://www.rand.org/content/dam/rand/pubs/research_memoranda/2009/RM727.1.pdf .
- 25. Kahn C.E., Genereaux B., Langlotz C.P. Conversion of Radiology Reporting Templates to the MRRT Standard. J. Digit. Imaging. 2015;28:528–536. doi: 10.1007/s10278-015-9787-3. [ DOI ] [ PMC free article ] [ PubMed ] [ Google Scholar ]
- 26. Becker G. Creating comparability among reliability coefficients: The case of Cronbach Alpha and Cohen Kappa. Psychol. Rep. 2000;87:1171. doi: 10.2466/pr0.2000.87.3f.1171. [ DOI ] [ PubMed ] [ Google Scholar ]
- 27. Cronbach L.J. Coefficient alpha and the internal structure of tests. Psychometrika. 1951;16:297–334. doi: 10.1007/BF02310555. [ DOI ] [ Google Scholar ]
- 28. [(accessed on 20 April 2021)]. Available online: https://recist.eortc.org/recist-1-1-2/
- 29. Marcovici P.A., Taylor G.A. Journal Club: Structured radiology reports are more complete and more effective than unstructured reports. AJR Am. J. Roentgenol. 2014;203:1265–1271. doi: 10.2214/AJR.14.12636. [ DOI ] [ PubMed ] [ Google Scholar ]
- 30. Ernst B.P., Strieth S., Katzer F., Hodeib M., Eckrich J., Bahr K., Rader T., Künzel J., Froelich M.F., Matthias C., et al. The use of structured reporting of head and neck ultrasound ensures time-efficiency and report quality during residency. Eur. Arch. Otorhinolaryngol. 2020;277:269–276. doi: 10.1007/s00405-019-05679-z. [ DOI ] [ PubMed ] [ Google Scholar ]
- 31. Goldberg-Stein S., Chernyak V. Adding Value in Radiology Reporting. J. Am. Coll. Radiol. 2019;16:1292–1298. doi: 10.1016/j.jacr.2019.05.042. [ DOI ] [ PubMed ] [ Google Scholar ]
- 32. Brady A.P. Radiology reporting-from Hemingway to HAL? Insights Imaging. 2018;9:237–246. doi: 10.1007/s13244-018-0596-3. [ DOI ] [ PMC free article ] [ PubMed ] [ Google Scholar ]
- 33. Weiss D.L., Langlotz C.P. Structured reporting: Patient care enhancement or productivity nightmare? Radiology. 2008;249:739–747. doi: 10.1148/radiol.2493080988. [ DOI ] [ PubMed ] [ Google Scholar ]
- 34. El Ayachy R., Giraud N., Giraud P., Durdux C., Giraud P., Burgun A., Bibault J.E. The Role of Radiomics in Lung Cancer: From Screening to Treatment and Follow-Up. Front. Oncol. 2021;11 doi: 10.3389/fonc.2021.603595. [ DOI ] [ PMC free article ] [ PubMed ] [ Google Scholar ]
- 35. Hepp T., Othman A., Liebgott A., Kim J.H., Pfannenberg C., Gatidis S. Effects of simulated dose variation on contrast-enhanced CT-based radiomic analysis for Non-Small Cell Lung Cancer. Eur. J. Radiol. 2020;124:108804. doi: 10.1016/j.ejrad.2019.108804. [ DOI ] [ PubMed ] [ Google Scholar ]
- 36. Xiong Z., Jiang Y., Che S., Zhao W., Guo Y., Li G., Liu A., Li Z. Use of CT radiomics to differentiate minimally invasive adenocarcinomas and invasive adenocarcinomas presenting as pure ground-glass nodules larger than 10 mm. Eur. J. Radiol. 2021;141:109772. doi: 10.1016/j.ejrad.2021.109772. [ DOI ] [ PubMed ] [ Google Scholar ]
- 37. Santone A., Brunese M.C., Donnarumma F., Guerriero P., Mercaldo F., Reginelli A., Miele V., Giovagnoni A., Brunese L. Radiomic features for prostate cancer grade detection through formal verification. La Radiol. Med. 2021;126:688–697. doi: 10.1007/s11547-020-01314-8. [ DOI ] [ PubMed ] [ Google Scholar ]
- 38. Granata V., Fusco R., Avallone A., De Stefano A., Ottaiano A., Sbordone C., Brunese L., Izzo F., Petrillo A. Radiomics-Derived Data by Contrast Enhanced Magnetic Resonance in RAS Mutations Detection in Colorectal Liver Metastases. Cancers. 2021;13:453. doi: 10.3390/cancers13030453. [ DOI ] [ PMC free article ] [ PubMed ] [ Google Scholar ]
- 39. Smedley N.F., Aberle D.R., Hsu W. Using deep neural networks and interpretability methods to identify gene expression patterns that predict radiomic features and histology in non-small cell lung cancer. J. Med. Imaging. 2021;8:031906. doi: 10.1117/1.JMI.8.3.031906. [ DOI ] [ PMC free article ] [ PubMed ] [ Google Scholar ]
- 40. Moreno S., Bonfante M., Zurek E., Cherezov D., Goldgof D., Hall L., Schabath M. A Radiogenomics Ensemble to Predict EGFR and KRAS Mutations in NSCLC. Tomography. 2021;7:154–168. doi: 10.3390/tomography7020014. [ DOI ] [ PMC free article ] [ PubMed ] [ Google Scholar ]
- 41. Granata V., Fusco R., Risi C., Ottaiano A., Avallone A., De Stefano A., Grimm R., Grassi R., Brunese L., Izzo F., et al. Diffusion-Weighted MRI and Diffusion Kurtosis Imaging to Detect RAS Mutation in Colorectal Liver Metastasis. Cancers. 2020;12:2420. doi: 10.3390/cancers12092420. [ DOI ] [ PMC free article ] [ PubMed ] [ Google Scholar ]
- 42. Reiner B.I. Strategies for Radiology Reporting and Communication. J. Digit. Imaging. 2014;27:1–6. doi: 10.1007/s10278-013-9656-x. [ DOI ] [ PMC free article ] [ PubMed ] [ Google Scholar ]
- 43. Hawkins C.M., Hall S., Zhang B., Towbin A.J. Creation and imple- mentation of department-wide structured reports: An analysis of the impact on error rate in radiology reports. J. Digit. Imaging. 2014;27:581–587. doi: 10.1007/s10278-014-9699-7. [ DOI ] [ PMC free article ] [ PubMed ] [ Google Scholar ]
- 44. Rosskopf A., Dietrich T.J., Hirschmann A., Buck F.M., Sutter R., Pfirrmann C. Quality Management in Musculoskeletal Imaging: Form, Content, and Diagnosis of Knee MRI Reports and Effectiveness of Three Different Quality Improvement Measures. Am. J. Roentgenol. 2015;204:1069–1074. doi: 10.2214/AJR.14.13216. [ DOI ] [ PubMed ] [ Google Scholar ]
- 45. Quattrocchi C.C., Giona A., Di Martino A.C., Errante Y., Scarciolla L., Mallio C.A., Denaro V., Zobel B.B. Extra-spinal inci- dental findings at lumbar spine MRI in the general population: A large cohort study. Insights Imaging. 2013;4:301–308. doi: 10.1007/s13244-013-0234-z. [ DOI ] [ PMC free article ] [ PubMed ] [ Google Scholar ]
- 46. ACoR Lung-Screening Reporting and Data System (LungRADS) Version 1.0. 2014. [(accessed on 20 April 2021)]. Available online: https://www.acr.org/-/media/ACR/Files/RADS/Lung-RADS/LungRADS_AssessmentCategories.pdf .
- 47. McKee B.J., Regis S.M., McKee A.B., Flacke S., Wald C. Performance of ACR Lung-RADS in a clinical CT lung screening program. J. Am. Coll Radiol. 2016;13:R25–R29. doi: 10.1016/j.jacr.2015.12.009. [ DOI ] [ PubMed ] [ Google Scholar ]
- 48. Aberle D. Implementing lung cancer screening: The US experience. Clin. Radiol. 2017;72:401–406. doi: 10.1016/j.crad.2016.12.003. [ DOI ] [ PubMed ] [ Google Scholar ]
- 49. ACoR Lung-Screening Reporting and Data System (LungRADS) Version 1.1. 2019. [(accessed on 20 April 2021)]. Available online: https://www.acr.org/-/media/ACR/Files/RADS/Lung-RADS/LungRADSAssessmentCategoriesv1-1.pdf?la=en .
- 50. ACoR ACR Lung-RADS-Update 1.1 2019. 2019. [(accessed on 20 April 2021)]. Available online: https://www.acr.org/-/media/ACR/Files/RADS/Lung-RADS/LungRADS-1-1-updates.pdf?la=en .
- 51. Grassi R., Miele V., Giovagnoni A. Artificial intelligence: A challenge for third millennium radiologist. Radiol. Med. 2019;124:241–242. doi: 10.1007/s11547-019-00990-5. [ DOI ] [ PubMed ] [ Google Scholar ]
- 52. Neri E., Coppola F., Miele V., Bibbolino C., Grassi R. Artificial intelligence: Who is responsible for the diagnosis? La Radiol. Med. 2020;125:517–521. doi: 10.1007/s11547-020-01135-9. [ DOI ] [ PubMed ] [ Google Scholar ]
- 53. Gatidis S., Heber S.D., Storz C., Bamberg F. Population-based imaging biobanks as source of big data. La Radiol. Med. 2016;122:430–436. doi: 10.1007/s11547-016-0684-8. [ DOI ] [ PubMed ] [ Google Scholar ]
- 54. Alberich-Bayarri Á., Hernández-Navarro R., Ruiz-Martínez E., García-Castro F., García-Juan D., Martí-Bonmatí L. Development of imaging biomarkers and generation of big data. La Radiol. Med. 2017;122:444–448. doi: 10.1007/s11547-017-0742-x. [ DOI ] [ PubMed ] [ Google Scholar ]
- 55. Carlos R.C., Kahn C., Halabi S. Data Science: Big Data, Machine Learning, and Artificial Intelligence. J. Am. Coll. Radiol. 2018;15:497–498. doi: 10.1016/j.jacr.2018.01.029. [ DOI ] [ PubMed ] [ Google Scholar ]
- 56. Morris M.A., Saboury B., Burkett B., Gao J., Siegel E.L. Reinventing Radiology: Big Data and the Future of Medical Imaging. J. Thorac. Imaging. 2018;33:4–16. doi: 10.1097/RTI.0000000000000311. [ DOI ] [ PubMed ] [ Google Scholar ]
- 57. Aphinyanaphongs Y. Big Data Analyses in Health and Opportunities for Research in Radiology. Semin. Musculoskelet. Radiol. 2017;21:32–36. doi: 10.1055/s-0036-1597255. [ DOI ] [ PubMed ] [ Google Scholar ]
- 58. Kansagra A.P., Yu J.-P., Chatterjee A.R., Lenchik L., Chow D., Prater A.B., Yeh J., Doshi A., Hawkins C.M., Heilbrun M.E., et al. Big Data and the Future of Radiology Informatics. Acad. Radiol. 2015;23:30–42. doi: 10.1016/j.acra.2015.10.004. [ DOI ] [ PubMed ] [ Google Scholar ]
Associated Data
This section collects any data citations, data availability statements, or supplementary materials included in this article.
- View on publisher site
- PDF (342.2 KB)
- Collections
Similar articles
Cited by other articles, links to ncbi databases.
- Download .nbib .nbib
- Format: AMA APA MLA NLM
Add to Collections
An official website of the United States government
Official websites use .gov A .gov website belongs to an official government organization in the United States.
Secure .gov websites use HTTPS A lock ( Lock Locked padlock icon ) or https:// means you've safely connected to the .gov website. Share sensitive information only on official, secure websites.
- Publications
- Account settings
- Advanced Search
- Journal List

Lung cancer staging: a physiological update †
Michael poullis, james mcshane, mathew shaw, steven woolley, michael shackcloth, richard page, neeraj mediratta.
- Author information
- Article notes
- Copyright and License information
Corresponding author. Consultant Cardiothoracic Surgeon, Liverpool Heart and Chest Hospital, Thomas Drive, Liverpool L14 3PE, UK. Tel: +44-151-2281616; fax: +44-151-2932254; e-mail: [email protected] (M. Poullis).
Presented at the 25th Annual Meeting of the European Association for Cardio-Thoracic Surgery, Lisbon, Portugal, 1–5 October 2011.
Received 2011 Sep 21; Revised 2011 Nov 26; Accepted 2011 Dec 7; Issue date 2012 Jun.
The tumour-node metastasis (TNM) classification system is anatomically based. We investigated whether the addition of simple physiological variables, age and body mass index (BMI), would affect survival curves, i.e. a composite anatomical and physiological staging system. We retrospectively analysed a prospectively validated thoracic surgery database ( n = 1981). Cox multivariate analysis was performed to determine possible significant factors. Kaplan–Meier survival curves were constructed with combined anatomical and physiological factors. Cox multivariate analysis revealed age ( P < 0.001) and BMI ( P = 0.01) as significant factors affecting survival. Receiver operating curve analysis determined cut-off levels for age of 67 and BMI of 27.6. A composite anatomical and physiological survival curve based on TNM for BMI > 27.6 and age < 67 was produced. Age and BMI criteria resulted in significantly different survival curves, for stage I ( P < 0.0001) and stage II ( P = 0.0032), but not for stage III ( P = 0.06). Neural network analysis confirmed the importance of BMI and age above cancer stage with regard to long-term survival. Combining age < 67, BMI > 27.6 and TNM anatomical classification results in very different estimated survival curves from the usual TNM system. Patients from stages I, II and III may have survival equivalent to a stage higher or lower depending on their age and BMI.
Keywords: Lung cancer, Staging, Physiology
INTRODUCTION
Staging of non-small-cell lung cancer is based on anatomical information with regard to tumour size, location and lymph node involvement and spread [ 1 ]. Factors other than stage can affect 5-year survival of lung cancer patients [ 2 – 4 ]. Using some of these factors may help predict high- and low-risk subgroups within a given stage. This may help select or indeed reject certain groups for neoadjuvant or adjuvant therapy.
We utilized a large prospective validated thoracic surgery database to evaluate simple, easily measured clinical factors that may have a significant contributing effect on long-term survival for a given stage.
Local institutional ethics board approval was obtained for this study.
Methodology
We retrospectively analysed a prospectively validated thoracic surgery database ( n = 1981). The demographics of the study population are shown in Table 1 . All non-anatomical resections were eliminated from the analysis.
Patient characteristics (operative and disease) in the study group
COPD: chronic obstructive pulmonary disease; IHD: ischemic heart disease; TIA/CVA: transient ischemic attack/cerebral vascular accident; DVT: deep vein thrombosis.
Categorical variables quoted as number (percentage) and continuous variables as median (inter-quartile range).
Staging was defined as pathological staging to eliminate bias by ‘better’ preoperative staging due to current multi-slice computed tomography (CT) and positron emission tomography (PET) scanning. Routine intraoperative mediastinal lymph node sampling has always been undertaken in our unit. PET scanning became routine for all patients 5 years ago in our unit. All patients with mediastinal lymph nodes enlarged by CT criteria or avid on PET were biopsied preoperatively via mediastinoscopy, mediastinotomy or endobronchial ultrasound.
Survival data for all patients are routinely obtained through the National Strategic Tracing Service, as previously described [ 5 ].
Benchmarking
We benchmarked our 5-year survival against the Seventh International Association for the Study of Lung Cancer (IALSC) [ 1 ] ( Supplementary material ).

Survival factor identification
Univariate analysis of all variables was performed first, with the objective of identifying significant factors for inclusion in a multivariate Cox regression model. Stepwise Cox proportional hazards regression analysis was used for the study population and each stage separately, as previous work has identified different factors as being significant for each stage [ 2 ] ( Supplementary material ). Entry criterion was P < 0.05, and removal criterion was P > 0.1. Cox risk-adjusted survival curves were created to demonstrate the effect of age and body mass index (BMI) on long-term survival (Fig. 1 ). The covariates were plotted at their mean.
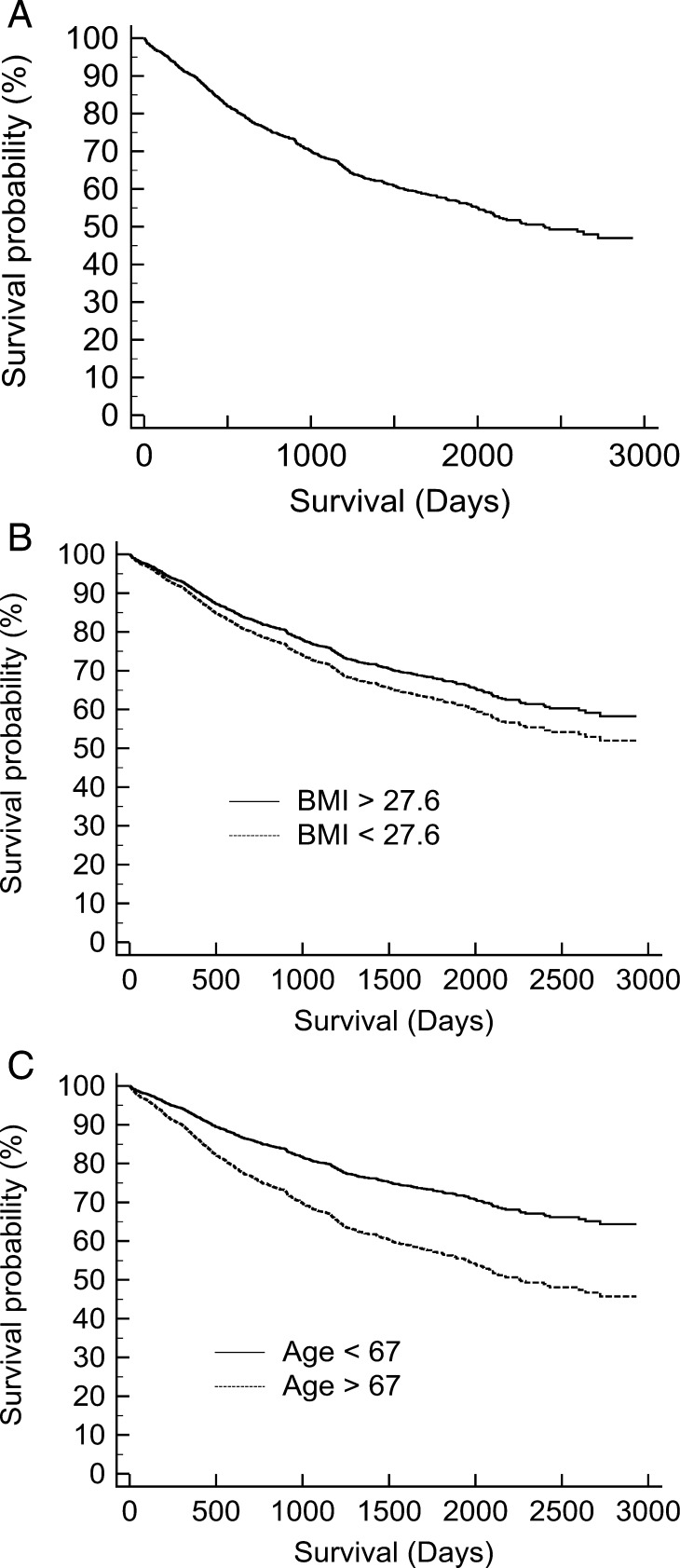
Cox risk-adjusted survival curves: ( A ) plotted at the mean of the covariates, ( B ) the effect of BMI and ( C ) the effect of age.
Receiver operating curve (ROC) analysis was performed to determine the cut-off values for Kaplan–Meier survival curves for any significant continuous variables.
Survival curves
Kaplan–Meier survival curves for all resection stages (I, II and IIIa) were constructed with combined anatomical and physiological factors (Fig. 2 ). The upper line of each stage contains patients with good (BMI > 27.6 and age < 67) prognostic factors identified above. The bottom line of each stage contains patients with poor (BMI < 27.6 and age > 67) prognostic factors identified above. Stage comparisons are shown in Fig. 3 .
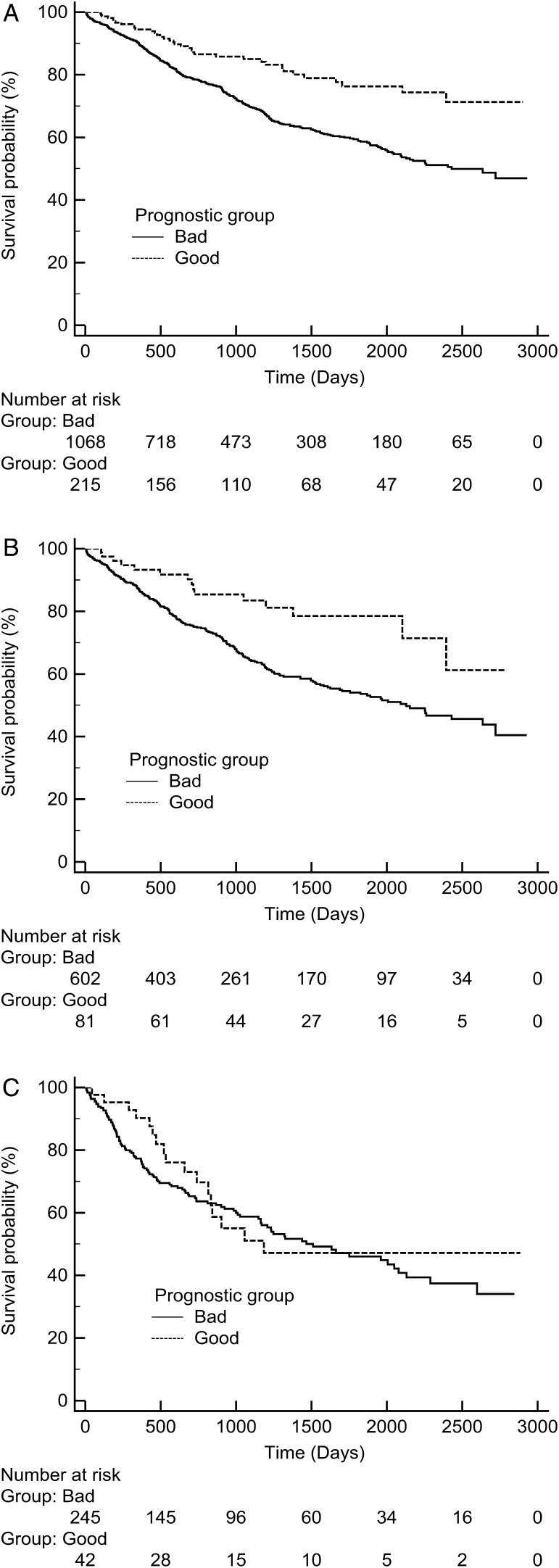
Kaplan–Meier survival curves for ( A ) stage I, ( B ) stage II and ( C ) stage IIIa. Good was defined as BMI > 27.6 and age < 67, and bad was defined as BMI < 27.6 and age > 67.
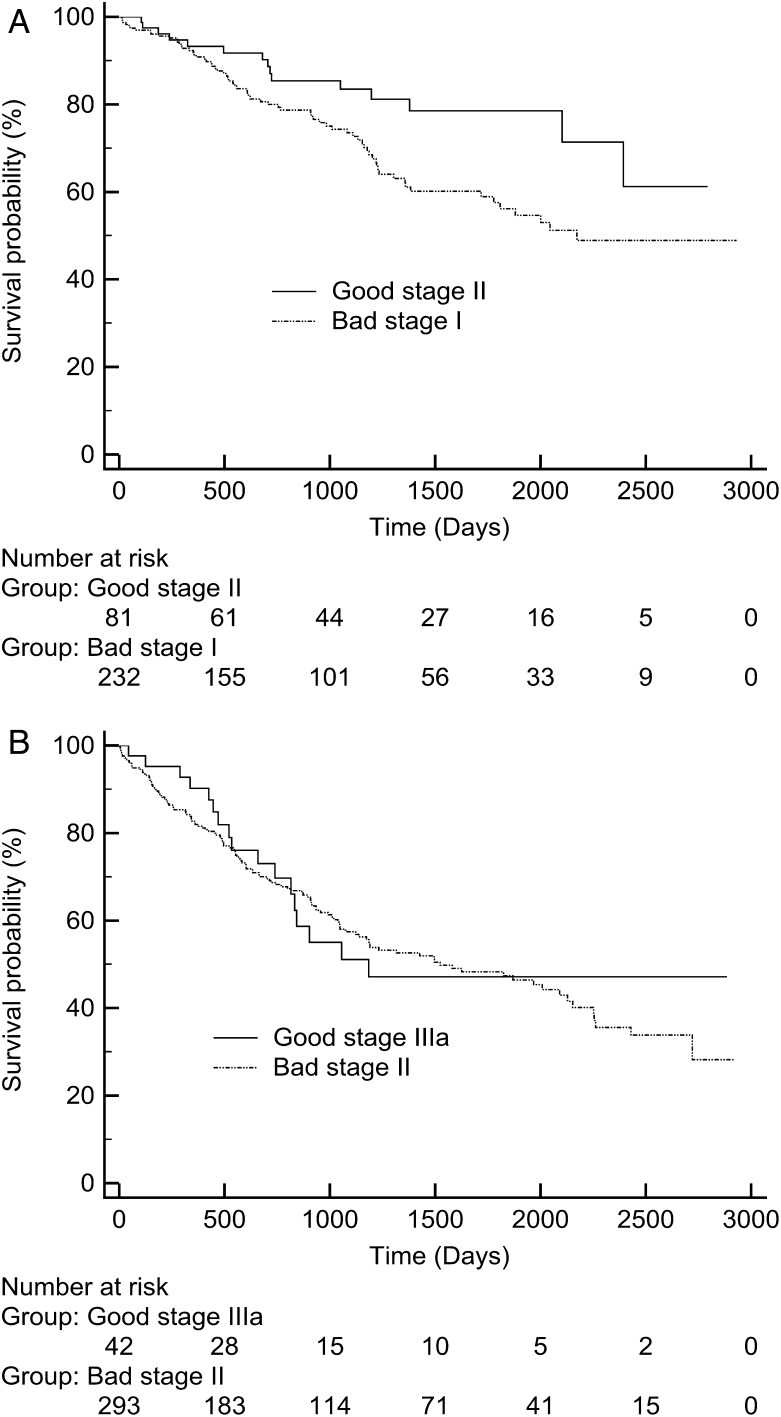
Comparison between ( A ) bad stage I and good II and ( B ) bad stage II and good IIIa. Good was defined as BMI > 27.6 and age < 67, and bad was defined as BMI < 27.6 and age > 67.
Adjuvant patients
Two hundred and eighty-seven patients had received adjuvant therapy. Analysis was performed with and without these patients included.
Neural network analysis
A neural network was then trained and run to create an ROC value to assess the network goodness-of-fit for predicting long-term survival to cross-validate the Cox regression analysis. All studied variables used in the Cox regression analysis were used as input variables for the neural network construction. The basis of this network is multilayer perceptions that have feed-forward back-propagation topology. The network consists of four layers as shown in Fig. 4 .
The input layer is of neurons into which variables were entered following their normalization through a process of standard rescaling (subtracting the mean and dividing by the standard deviation).
Two hidden layers composed of neurons in which computation and differential weighing of the different variables are performed, using the hyperbolic tangent function.
The output layer is two neurons into which the end result is entered (alive or dead), via the Softmax function.
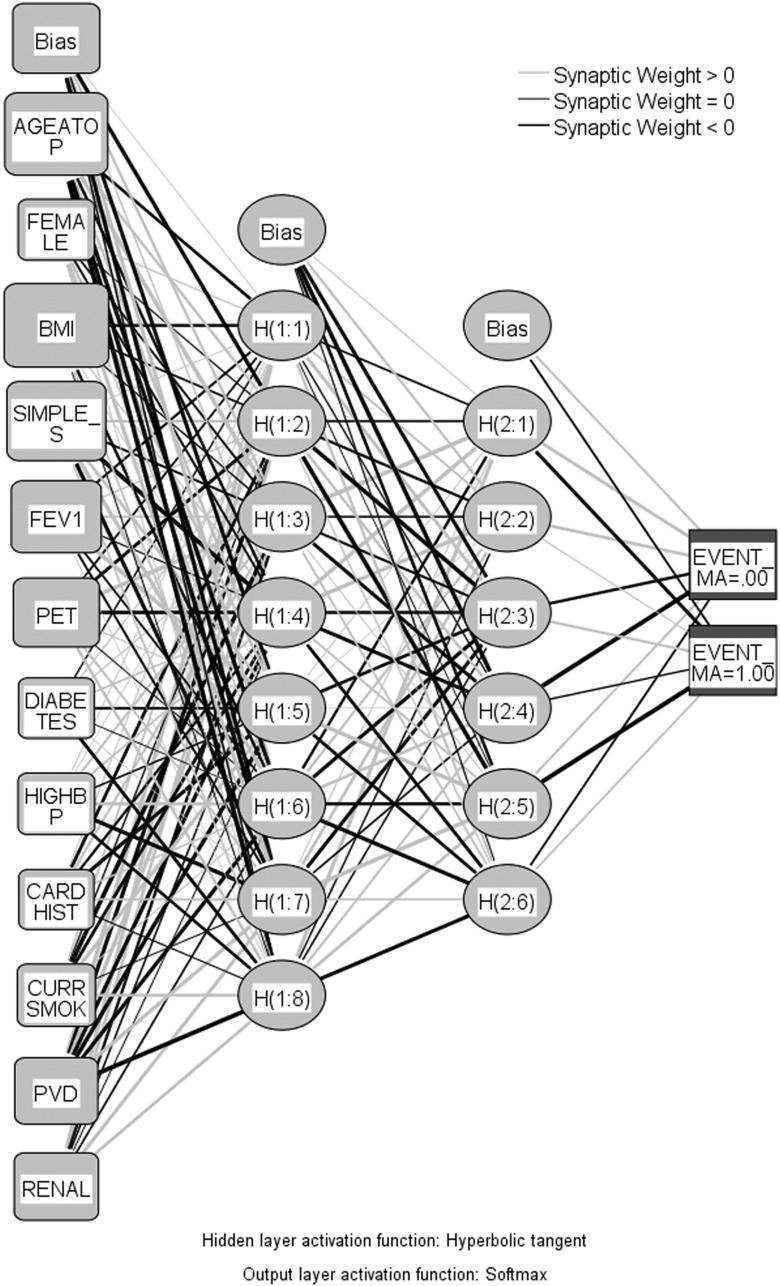
Neural network utilized for predicting long-term survival. Key: AGEATOP: age at operation; FEMALE: sex; BMI: body mass index; simple stage: TNM stage; FEV1: forced expiratory volume in 1 s; PET: positron emission tomography; DIABETES: diabetes; HIGHBP: high blood pressure; CARDHIST: previous myocardial infarct or angina; CURRSMOK: current smoker; PVD: peripheral vascular disease; RENAL: renal failure, dialysis or creatinine > 200 μmol/l.
Normalized importance output
This was used to determine factors that are important in predicting long-term survival post-resection for potentially curative non-small-cell lung cancer (Fig. 5 ).
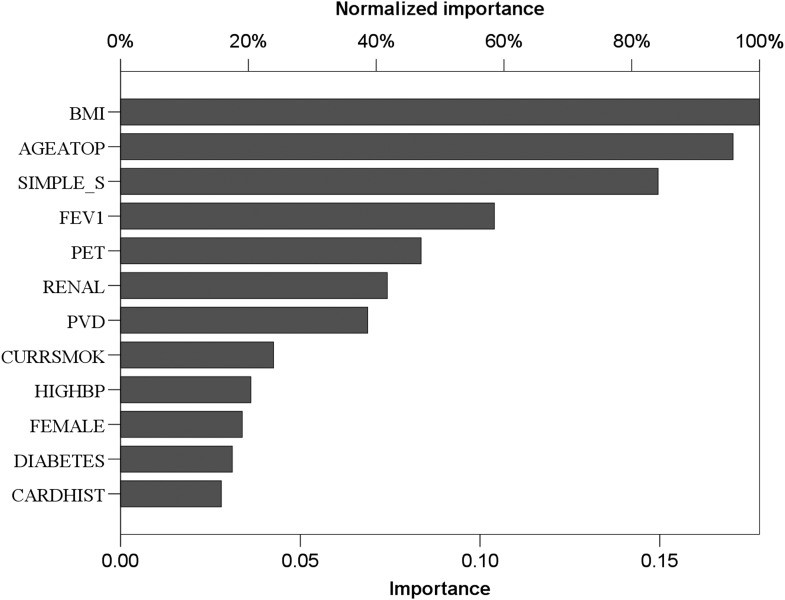
Normalized importance output from neural network analysis. For key, see legend to Fig. 4 .
Statistical software
All statistical analyses other than the neural network were performed with MedCalc for Windows (version 11.4.2.0, MedCalc Software, Mariakerke, Belgium). The neural network analysis was performed with SPSS (version 17.0 for Windows; SPSS, Inc., Chicago, IL, USA).
One hundred percent patient follow-up was obtained. Overall institutional in hospital mortality was 1.6% for all lobectomy and pneumonectomy resections.
Benchmarking failed to reveal any significant differences between our institution's stage survival and the Seventh IALSC results ( Supplementary material ).
Cox analysis
Cox multivariate analysis (Table 2 ) revealed age ( P < 0.001) and BMI ( P = 0.01) as significant factors affecting survival. ROC analysis determined cut-off levels for age of 67 (sensitivity 57.3%, specificity 56.9%) and BMI of 27.6 (sensitivity 72.8%, specificity 36.5%).
Cox regression analysis of factors affecting long-term survival (all stages)
Cox risk-adjusted survival curves demonstrated the significant effect of age on long-term survival (Fig. 1 ).
Kaplan–Meier curves
A composite anatomical and physiological Kaplan–Meier survival curve based on tumour-node metastasis (TNM), BMI (cut-off 27.6) and age (cut-off 67) was produced. Good was defined as BMI > 27.6 and age < 67, and bad was defined as BMI < 27.6 and age > 67. Age and BMI criteria resulted in significantly different survival curves, for stage I ( P < 0.0001) and stage II ( P = 0.0032), but not for stage III ( P = 0.06) (Fig. 3 ).
Kaplan–Meier curves comparing stages I and II, and II and IIIa are shown in Fig. 3 . It can be seen that stage overlap occurs, making survival prediction based on stage alone potentially erroneous.
Adjuvant therapy
Inclusion and exclusion of patients who had received adjuvant therapy from the analysis did not affect the above results (data not shown).
The normalized predictive values of the neural network (Fig. 5 ) identified age and BMI were of higher predictive value than the TNM stage, with regard to survival.
Simple, easily measured clinical variables such as age and BMI have significant effects on the survival of patients with non-small-cell lung cancer. This may have implications for survival curve analysis in neoadjuvant and adjuvant therapy trials.
The use of ROC curves to identify cut-off levels for the creation of the Kaplan–Meier survival curves was to aid graphical representation of the proposed concepts and for proof-of-principle only. In clinical practice, age and BMI are continuous variables and should be treated as such. To predict survival based on these variables, the parameter estimates obtained from the Cox regression analysis should be used, as described previously [ 6 ].
All non-anatomical resections were eliminated from this analysis. Previous work has demonstrated that wedge resections were an independent factor predicting poor outcomes [ 2 , 7 , 8 ].
The choice of age and BMI as segregation variables was based only on three facts. First, the data set, despite its large size, is too small to subdivide patients into strata defined by all the significant factors identified by Cox regression analysis. Second, neural network analysis identified age and BMI as having higher importance in determining survival than TNM stage. Third, we have demonstrated via propensity matching that BMI is a significant determinant of long-term survival (Shackcloth et al. , in press).
Combining age < 67, BMI > 27.6 and TNM anatomical classification results gives very different estimated survival curves from the usual TNM system. Patients from stages I, II and IIIa may have survival equivalent to a stage higher or lower depending on their age and BMI. This may have a significant effect when considering adjuvant therapy.
Quality control is an important part of modern thoracic surgery practice, with benchmarking against other units an important part of this [ 9 ]. The addition of simple clinical variables to the current TNM staging system may allow some of the contributing variables that exist between different institutions to be accounted for.
A number of molecular biology markers that are associated with a good or bad prognosis have been identified [ 10 , 11 ]; however, these remain research tools and are not universally available, unlike the simple variables such as age and BMI.
Entry into oncologic trials is usually histology- and stage-based [ 12 ]; however, we have shown that simple physiological variables have a significant effect on long-term survival. The Adjuvant Lung Cancer Trial Collaborative Group studies include age and sex, amongst other variables, but do not include BMI as a contributing factor, despite Cox regression and neural network analyses demonstrating it to be a significant factor.
We were unable to include all other factors identified by regression analysis due to the limited size of our database, despite it containing nearly 2000 resections. Neural networks remain contentious due to their ‘black box’ technology; however, t -test results from the neural network normalized importance indicate that we have chosen the two most important variables. The aim of this concept paper was simplicity combined with clinical ease of applicability.
Patients who received adjuvant therapy potentially could have skewed the data; however, when analysed with or without these patients, the same results were reached. This could have been a source of bias as adjuvant patients tend to be younger and be less likely to be wasted, i.e. a BMI > 27.6, due to their selection usually based on performance status, of an appropriate TNM stage.
Adjuvant therapy increases survival by ∼5% [ 12 ], whereas patient physiological variables alter survival by 15%. Identification of patient groups that do better or worse than average does not correlate with the response rate to neoadjuvant or adjuvant therapy. Further work is needed to identify for a given stage which physiological group(s) will benefit most from oncological therapy, which is currently anatomically selected via the TNM system and performance status guided.
In conclusion, the addition of simple physiological variables to the anatomical TNM staging system identifies large variations in survival for a given stage. Overlap in survival between stages occurs between ‘good’ and ‘bad’ patient physiological parameters.
SUPPLEMENTARY MATERIAL
Supplementary material is available at ICVTS online.
Conflict of interest: none declared.
APPENDIX. CONFERENCE DISCUSSION
Dr G. Cardillo (Rome, Italy) : This well-written, quality-content paper coming from a well-known, high-volume UK institution, the Liverpool Heart and Chest Hospital, reports an analysis of 1,981 patients from the thoracic database with an impressive 100% follow-up.
I have two questions, the first regarding age and the second regarding BMI. Since the paper by Sugarbaker in 1999 which stated age to be a risk factor for death after thoracotomy, a large number of papers have shown the safety of lobectomy in patients older than 80 years. Furthermore, the age limit that you have chosen, 67.6 years, has a very low impact on our practice, since epidemiologic data have shown that 70% of lung cancers are diagnosed over 65 years and the median age is 71.4. Could you please comment on how this age limit could impact on our practice? Regarding BMI, I have seen that the limit that you have chosen is 27.6, but overweight is over 25 and obesity is over 30. What do you suggest, that we have to change our vision of BMI?
Dr Poullis : In the manuscript it's carefully stated in the discussion. The reason I used the cut-off values was clearly for demonstration of the concept I was trying to present. It's not for clinical practice where you become 70 and suddenly you can have a different operation or treatment. That's not the game. You get the same treatment surgically no matter what your age or your BMI. Now, because we can depict very different survival curves in these two subsets of patients, it then has implications. Who should get the chemotherapy? Is it the bad group or is it the good group? We don't know. When I looked at Agregado, I think it is, his latest adjuvant chemotherapy trials don't include BMI. Age is included. So I'm not trying to alter the surgical therapy. I'm just saying that it's not as simple at the moment where, if you have a fixed stage and you're fit, you get chemo, and if you're the wrong stage, you don't get chemo.
The ROC curves I showed were purely for graphical representation of the concept. That's not how it's practised clinically. I made that clear in the discussion of the paper. It's purely to show you the curves. I show you the Cox regression and the P-value of surgeons, but if I show you two Kaplan-Meier curves completely apart, the fact that you do better in stage II than you do in stage I, depending on your risk factors, is easier to see graphically than on a Cox. You are right in your point.
Dr M. Dusmet (London, United Kingdom) : Looking at this and the previous presentation from your centre, there are some intriguing things that I'd like to hear your thoughts on. First of all, if I'm correct, both papers were based upon exactly the same database?
Dr Poullis : Yes.
Dr Dusmet : And yet the operative mortality in the first paper is 2.5% and in yours it's just over 1.5%. That's my first question.
Dr Poullis : It's exactly the same database, but the timeframe that the data is taken from is different between the two studies. The two projects we did actually were completely independent, as in a typical department, not talking to each other. That's why the total number of patients is different between the two. Also, I have excluded all people with residual disease in my dataset, which wasn't done in the first paper.
Dr Dusmet : The second question leads on from what Giuseppe was just saying. What you've shown when you put the data of these two papers together is that when your BMI is higher, you have a better chance of survival, but, as Giuseppe just pointed out, statistically your chances of being older are also greater, and, secondly, you've demonstrated the chances of being diabetic and having renal impairment are greater. Therefore, by the time you're older and you have renal impairment and you have diabetes, the likelihood of getting adjuvant chemotherapy is virtually nil because the oncologists do not like to kill patients who have been offered curative surgery. So your thoughts on that, please.
Dr Poullis : Well, you are exactly right. My point about what the oncologists do is based on stage and performance status. That's the only two things that go through their minds. You have to be at the right stage and fit. And I'm saying, well, actually, it's more complicated than that. You can be at the wrong stage and have a terrible survival, which might mean you need adjuvant therapy. It might not. We don't know from the data. That's the flaw in the adjuvant data. They looked at age only and they have looked at a lot of other factors, but they didn't look at BMI. When I looked through the papers, I couldn't find BMI as a matching factor in any of the adjuvant lung cancer trials.
Dr P. Van Schil (Antwerp, Belgium) : An important factor that is often forgotten, even in the adjuvant therapy trials, is the smoking status of the patients. In our series this is a major prognostic factor. Did you look at it, as it can be an important factor influencing outcome.
Dr Poullis : Yes, I have looked at it, and that's hopefully going to be work that I'll be presenting soon, if I get it accepted. We looked at people who are still smoking, people who are ex-smokers, and people who have never smoked who have lung cancer. Dr Van Schil : And those who resumed smoking after the operation?
Dr Poullis : Well, we counted those as continuous smokers. Liverpool has quite a few. It's a dedicated smoking city. It turns out that if you've never smoked or you're still smoking, your survival is way, way worse than if you stop smoking.
Dr L. Spaggiari (Milan, Italy) : I have a brief comment. We analysed a large series of patients who underwent pneumonectomy according to several parameters, including BMI, and we agree with your results. There is a strong correlation between survival in pneumonectomized patients and BMI.
Dr Poullis : Yes. The only argument for the cut-off for the BMI in the paper was that in the literature everyone uses 30, but in our database it wasn't 30. It was reasonably close. So I didn't know, do I just use 30, which everyone else is using, or do I use what our database showed? But really, at the end of the day, it's just for demonstration of the point that BMI is important, age is important, and they are not factors usually considered. It's usually if they're really old, no adjuvant therapy, but there's no other consideration in any of the decisions.
Dr Spaggiari : We need to study BMI.
- 1. Chansky K, Sculier JP, Crowley JJ, Giroux D, Van Meerbeeck J, Goldstraw P. The International Association for the Study of Lung Cancer Staging Project: prognostic factors and pathologic TNM stage in surgically managed non-small cell lung cancer. J Thorac Oncol. 2009;4:792–801. doi: 10.1097/JTO.0b013e3181a7716e. doi:10.1097/JTO.0b013e3181a7716e . [ DOI ] [ PubMed ] [ Google Scholar ]
- 2. Fontaine E, McShane J, Carr M, Shackcloth M, Mediratta N, Page R, et al. Does positron emission tomography scanning improve survival in patients undergoing potentially curative lung resections for non-small-cell lung cancer? Eur J Cardiothorac Surg. 2011;40:642–6. doi: 10.1016/j.ejcts.2010.12.053. [ DOI ] [ PubMed ] [ Google Scholar ]
- 3. Yano M, Sasaki H, Moriyama S, Kawano O, Hikosaka Y, Fujii Y. Prognostic factors of pathologic stage IB non-small cell lung cancer. Ann Thorac Cardiovasc Surg. 2011;17:58–62. doi: 10.5761/atcs.cr.09.01481. doi:10.5761/atcs.cr.09.01481 . [ DOI ] [ PubMed ] [ Google Scholar ]
- 4. Zhou W, Christiani DC. East meets West: ethnic differences in epidemiology and clinical behaviors of lung cancer between East Asians and Caucasians. Chin J Cancer. 2011;30:287–92. doi: 10.5732/cjc.011.10106. doi:10.5732/cjc.011.10106 . [ DOI ] [ PMC free article ] [ PubMed ] [ Google Scholar ]
- 5. Fontaine E, McShane J, Page R, Shackcloth M, Mediratta N, Carr M, et al. Aspirin and non-small cell lung cancer resections: effect on long-term survival. Eur J Cardiothorac Surg. 2010;38:21–6. doi: 10.1016/j.ejcts.2010.01.015. doi:10.1016/j.ejcts.2010.01.015 . [ DOI ] [ PubMed ] [ Google Scholar ]
- 6. Fontaine E, McShane J, Carr M, Shachcloth M, Mediratta N, Page R, et al. Should we operate on microscopic N2 non small cell lung cancer? Interact CardioVasc Thorac Surg. 2011;12:956–61. doi: 10.1510/icvts.2010.255323. doi:10.1510/icvts.2010.255323 . [ DOI ] [ PubMed ] [ Google Scholar ]
- 7. Chamogeorgakis T, Ieromonachos C, Georgiannakis E, Mallios D. Does lobectomy achieve better survival and recurrence rates than limited pulmonary resection for T1N0M0 non-small cell lung cancer patients? Interact CardioVasc Thorac Surg. 2009;8:364–72. doi: 10.1510/icvts.2008.178947. doi:10.1510/icvts.2008.178947 . [ DOI ] [ PubMed ] [ Google Scholar ]
- 8. Landreneau RJ, Sugarbaker DJ, Mack MJ, Hazelrigg SR, Luketich JD, Fetterman L, et al. Wedge resection versus lobectomy for stage I (T1 N0 M0) non-small-cell lung cancer. J Thorac Cardiovasc Surg. 1997;113:691–98. doi: 10.1016/S0022-5223(97)70226-5. doi:10.1016/S0022-5223(97)70226-5 . [ DOI ] [ PubMed ] [ Google Scholar ]
- 9. Brunelli A, Berrisford RG, Rocco G, Varela G. The European Thoracic Database project: composite performance score to measure quality of care after major lung resection. Eur J Cardiothorac Surg. 2009;35:769–74. doi: 10.1016/j.ejcts.2009.01.037. doi:10.1016/j.ejcts.2009.01.037 . [ DOI ] [ PubMed ] [ Google Scholar ]
- 10. Kadara H, Lacroix L, Behrens C, Solis L, Gu X, Lee JJ, et al. Identification of gene signatures and molecular markers for human lung cancer prognosis using an in vitro lung carcinogenesis system. Cancer Prev Res (Phila) 2009;2:702–11. doi: 10.1158/1940-6207.CAPR-09-0084. doi:10.1158/1940-6207.CAPR-09-0084 . [ DOI ] [ PMC free article ] [ PubMed ] [ Google Scholar ]
- 11. Zhu CQ, Pintilie M, John T, Strumpf D, Shepherd FA, Der SD, et al. Understanding prognostic gene expression signatures in lung cancer. Clin Lung Cancer. 2009;10:331–40. doi: 10.3816/CLC.2009.n.045. doi:10.3816/CLC.2009.n.045 . [ DOI ] [ PubMed ] [ Google Scholar ]
- 12. Arriagada R, Dunant A, Pignon JP, Bergman B, Chabowski M, Grunenwald D, et al. Long-term results of the international adjuvant lung cancer trial evaluating adjuvant cisplatin-based chemotherapy in resected lung cancer. J Clin Oncol. 2010;28:35–42. doi: 10.1200/JCO.2009.23.2272. doi:10.1200/JCO.2009.23.2272 . [ DOI ] [ PubMed ] [ Google Scholar ]
Associated Data
This section collects any data citations, data availability statements, or supplementary materials included in this article.
Supplementary Materials
- View on publisher site
- PDF (267.7 KB)
- Collections
Similar articles
Cited by other articles, links to ncbi databases.
- Download .nbib .nbib
- Format: AMA APA MLA NLM
Add to Collections
Enhanced Lung Cancer Detection and TNM Staging Using YOLOv8 and TNMClassifier: An Integrated Deep Learning Approach for CT Imaging
Ieee account.
- Change Username/Password
- Update Address
Purchase Details
- Payment Options
- Order History
- View Purchased Documents
Profile Information
- Communications Preferences
- Profession and Education
- Technical Interests
- US & Canada: +1 800 678 4333
- Worldwide: +1 732 981 0060
- Contact & Support
- About IEEE Xplore
- Accessibility
- Terms of Use
- Nondiscrimination Policy
- Privacy & Opting Out of Cookies
A not-for-profit organization, IEEE is the world's largest technical professional organization dedicated to advancing technology for the benefit of humanity. © Copyright 2024 IEEE - All rights reserved. Use of this web site signifies your agreement to the terms and conditions.
Lung cancer staging: a concise update
Affiliations.
- 1 Dept of Thoracic Surgery, Hospital Universitari Mutua Terrassa, University of Barcelona, Barcelona, Spain.
- 2 Network of Centres for Biomedical Research in Respiratory Diseases (CIBERES) Lung Cancer Group, Barcelona, Spain.
- 3 Dept of Morphological Sciences, School of Medicine, Universitat Autònoma de Barcelona, Barcelona, Spain.
- 4 Dept of Respiratory Diseases, University Hospitals, KU Leuven, Leuven, Belgium.
- 5 Centre of Imaging Diagnosis, Radiology Dept, Hospital Clínic, University of Barcelona, Barcelona, Spain.
- 6 Dept of Pathology, Memorial Sloan Kettering Cancer Center, New York, NY, USA.
- PMID: 29700105
- DOI: 10.1183/13993003.00190-2018
Diagnosis and clinical staging of lung cancer are fundamental to planning therapy. The techniques for clinical staging, i.e anatomic and metabolic imaging, endoscopies and minimally invasive surgical procedures, should be performed sequentially and with an increasing degree of invasiveness. Intraoperative staging, assessing the magnitude of the primary tumour, the involved structures, and the loco-regional lymphatic spread by means of systematic nodal dissection, is essential in order to achieve a complete resection. In resected tumours, pathological staging, with the systematic study of the resected specimens, is the strongest prognostic indicator and is essential to make further decisions on therapy. In the present decade, the guidelines on lung cancer staging of the American College of Chest Physicians and the European Society of Thoracic Surgeons are based on the best available evidence and are widely followed. Recent advances in the classification of the adenocarcinoma of the lung, with the definition of adenocarcinoma in situ , minimally invasive adenocarcinoma and lepidic predominant adenocarcinoma, and the publication of the eighth edition of the tumour, node and metastasis classification of lung cancer, have to be integrated into the staging process. The present review complements the latest guidelines on lung cancer staging by providing an update of all these issues.
Copyright ©ERS 2018.
Publication types
- Adenocarcinoma / classification*
- Adenocarcinoma / diagnostic imaging*
- Adenocarcinoma / therapy
- Bronchoscopy
- Diagnostic Imaging / methods
- Disease Management
- Endosonography
- Lung / pathology
- Lung Neoplasms / classification*
- Lung Neoplasms / diagnostic imaging*
- Lung Neoplasms / therapy
- Minimally Invasive Surgical Procedures
- Neoplasm Staging
- Practice Guidelines as Topic
- Randomized Controlled Trials as Topic

IMAGES
COMMENTS
The seventh edition of the non-small cell lung cancer (NSCLC) TNM staging system was developed by the International Association for the Staging of Lung Cancer (IASLC) Lung Cancer Staging Project by a coordinated international effort to develop data-derived TNM classifications with significant surviv …
Nicholson AG, Chansky K, Crowley J, Beyruti R, Kubota K, Turrisi A, et al. The International Association for the Study of Lung Cancer Lung Cancer Staging Project:Proposals for the Revision of the Clinical and Pathologic Staging of Small Cell Lung Cancer in the Forthcoming Eighth Edition of the TNM Classification for Lung Cancer.
The iaslc lung cancer staging project: Proposals for the revision of the tnm stage groupings in the forthcoming (seventh) edition of the tnm classification of malignant tumours. ... Quality of life. Medical research council lung cancer working party. Br J Cancer. 1993;68(6):1157-1166. doi: 10.1038/bjc.1993.497. [PMC free article ...
Lung cancer is the leading cause of cancer-related death in the United States and accounts for more deaths than colorectal, breast, prostate, and pancreatic cancers combined. The eighth edition of the tumor-node-metastasis staging system (TNM-8), which has been accepted by the Union for International Cancer Control and the American Joint Committee on Cancer based on proposals from the ...
We hope that this paper will serve as a bridge between clinicians and basic researchers and aid in our understanding of lung cancer. ... Atlas of lung cancer staging. T factor is determined by size, location, invasion, and number of primary tumors. ... This research was supported by Basic Science Research Program through the National Research ...
Lung cancer is the leading cause of cancer morbidity and mortality in men, whereas in women, it ranks third for incidence after breast and colorectal cancer, and second for mortality after breast cancer . The incidence and mortality rates are roughly twice as high in men than in women, although the male-to-female ratio varies widely across regions.
Staging of non-small-cell lung cancer is based on anatomical information with regard to tumour size, location and lymph node involvement and spread . Factors other than stage can affect 5-year survival of lung cancer patients [2-4]. Using some of these factors may help predict high- and low-risk subgroups within a given stage.
This paper introduces an advanced method for lung cancer subtype classification and detection using the latest version of YOLO, tailored for the analysis of CT images. Given the increasing mortality rates associated with lung cancer, early and accurate diagnosis is crucial for effective treatment planning. The proposed method employs single-shot object detection to precisely identify and ...
Lung cancer staging is necessary for prognostication, to inform treatment options, and to allow accurate representation in clinical trials. Staging also separates operable from inoperable disease.
Diagnosis and clinical staging of lung cancer are fundamental to planning therapy. The techniques for clinical staging, i.e anatomic and metabolic imaging, endoscopies and minimally invasive surgical procedures, should be performed sequentially and with an increasing degree of invasiveness. Intraoperative staging, assessing the magnitude of the primary tumour, the involved structures, and the ...